7 January 2025
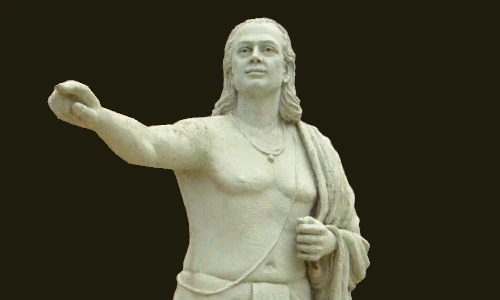
In this article we solve a non-linear curve fitting problem using the SciPy library. SciPy is especially well-suiting to solving this type of problem, as it includes a variety of functions for fitting and optimizing non-linear functions.
Along the way, we illustrate how to use SciPy's curve_fit
and minimize
functions for this type of task. In addition, we look at some good practices to apply when solving this type of problem, including:
- Using different criteria for defining the best fit, such as sum of squared differences and largest absolute error.
- Examining use of the
full_output
option when using thecurve_fit
function, to get more information about the result. - Examining the
success
andmessage
values of theminimize
function result to ensure that the solver converges correctly. - Trying a variety of
minimize
solution methods to see which one works best in our specific situation. - Fine-tuning the solution by changing the convergence tolerance.
4 December 2024

In this article we pursue an ambitious goal: Create an entire, non-trivial optimization model using Artificial Intelligence (AI).
Our approach mimics a user who is familiar with the situation, and has some experience with formulating optimization models, but is not familiar with implementing an optimization model in Python code. We want the AI to do all the coding for us.
It's not surprising that others precede us in pursuing this goal. For example:
We provide an overview of a system which uses artificial intelligence and database techniques to help a knowledgeable user formulate large linear programs. The system automates many of the tedious processes associated with large-scale modeling […]
Initially, the system will be most suitable for expert users; eventually we hope that it will become intelligent enough to help managers or students with a minimal exposure to linear programming techniques.
"An intelligent system for formulating linear programs", Murphy & Stohr
What may be surprising is that Murphy & Stohr's article was published in 1985, almost 40 years ago. The AI tool they used, a Prolog rules-based expert system, is very different to the Large Language Model (LLM) AI tools currently in vogue. Murphy & Stohr report some success in using their AI system, though their approach did not become commonly used. Nonetheless, their goal then was much the same as it is for us now.
We report our experience of using Copilot to write a model for us. Rather than presenting a verbatim transcript, which is very long, we focus on what went well and what didn't go well as the model evolves. We also summarize general lessons from the process.
14 November 2024
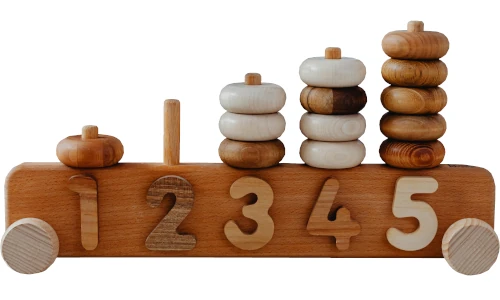
We replicate a model by Erwin Kalvelagen at Yet Another Math Programming Consultant (YAMPC), Sorting using a MIP model. Erwin explores the run time behaviour of the CPLEX, HiGHS, and Xpress solvers for this feasibility problem, using an objective function that has a constant value of zero.
Professor Paul Rubin explores the same model in Solver parameters matter, using the CPLEX and Xpress solvers, finding that small tweaks to the solver options can have large impacts on relative solver performance.
In this article, we assess the impact of using an alternative objective function in the same model. The idea is to give the HiGHS solver greater traction while working through the solution space, hopefully helping it to solve the model faster. We've found this technique to be useful for some other models – will it help in this situation?
26 October 2024
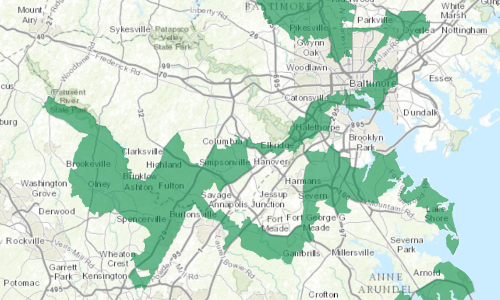
Our previous article implores academic researchers to publish data and code to accompany their papers. We use an example where academic publishing has been done well: with papers about creating voting districts accompanied by data and models available on Github.
On a related topic, Alireza Soroudi has published an article on LinkedIn describing a Gerrymandering using Constraint Programming model. Dr. Soroudi's model has the specific objective of showing how electoral district boundaries can be manipulated to advantage a party, group, or socioeconomic class within the constituency. As Dr. Soroudi says:
Gerrymandering – the crafty art of redrawing electoral districts to tip the scales in favor of a particular party – is as intriguing as it is controversial.
In this article, we take a simple approach to modifying a redistricting model. We add a requirement to our model that could be interpreted as either:
- The laudable goal of grouping together "communities of interest" – a common requirement when designing voting districts; or
- A nefarious attempt to manipulate the electoral outcome by gerrymandering.
Gerrymandering is the opposite of the model's purpose in our previous article. But, as model designers, we need to be aware that we don't always control the purposes to which decision makers apply our models and decision makers don't always understand the implications of small changes to a model.
2 October 2024

Academic research papers can be a valuable source of material for creating and improving real world optimization models. But, as we have commented previously, we wish that academics would publish working code and data to accompany their papers.
In many papers, the mathematical formulations are poorly defined and hard to understand – often they are just plain wrong. But even when the math is correct, the lack of code and data accompanying most academic papers makes them difficult and time-consuming to replicate, which greatly undermines their value.
Some academic institutions understand the importance and value of publishing data and code, for example:
Reproducible science requires not just sharing the paper and the data, but the methods as well. When those methods are partly or entirely captured by computer code, sharing that code is a powerful step towards making your work fully reproducible. Not only does this allow verification and detailed exploration of your findings, but it also facilitates further research; well-written code can be re-used and even built upon, as a scientific product in and of itself.
Publishing code and software, Utrecht University, The Netherlands
But such a sentiment is an exception rather than the norm.
In this article:
- Firstly, we briefly look at some reasons why academics might be reluctant to publish their data and code.
- Then we replicate, modify, and explore a published model that has been done well, with the data and program code publicly available.
12 September 2024
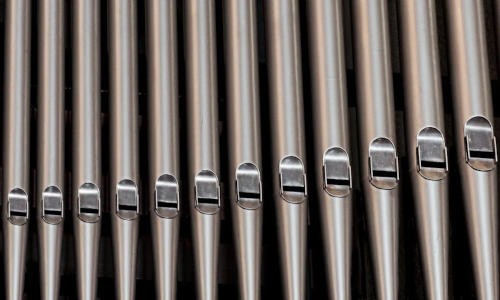
We complete an exploration of methods to solve our device rack positioning problem. The methods are:
- Model 1. Enumerate all possible position orders.
- Model 2. Search randomly for a specified time.
- Model 3. Local search around random starting positions.
- Model 4. Constraint programming using OR-Tools.
- Model 5. Mixed integer linear programming using Pyomo.
Of the models we've looked at so far, the constraint programming method works best. Model 4 quickly finds optimal solutions for all cases except the largest (24 device) case. Even then, its solution for the 24 device case is probably optimal.
In this article, we develop Model 5, which formulates the situation as a Mixed Integer Linear Program (MILP) in Pyomo. Will this method work as well as constraint programming, or perhaps even better?
26 August 2024

We continue our exploration of methods to solve our device rack positioning problem. The methods are:
- Model 1. Enumerate all possible position orders.
- Model 2. Search randomly for a specified time.
- Model 3. Local search around random starting positions.
- Model 4. Constraint programming using OR-Tools.
- Model 5. Mixed integer linear programming using Pyomo.
The previous articles describe the enumeration and parallel random search methods. Enumeration finds optimal solutions for the small data sets, but performs poorly for larger data sets. The random search method finds good solutions for the larger data sets, but those solutions are unlikely to be optimal. Using a more focussed local search method, we improved the solutions even further.
In this article, we develop Model 4 which formulates the situation as a Constraint Programming problem and solves it using the CP-SAT solver in OR-Tools. Perhaps this more sophisticated method will enable us to find optimal solutions for all of our data sets?
13 August 2024

We continue our exploration of methods to solve our device rack positioning problem. The methods are:
- Model 1. Enumerate all possible position orders.
- Model 2. Search randomly for a specified time.
- Model 3. Local search around random starting positions.
- Model 4. Constraint programming using OR-Tools.
- Model 5. Mixed integer linear programming using Pyomo.
The previous article describes Model 2, which uses a parallel random search method to find good solutions. Model 2 works surprisingly well, even for the larger data sets, finding solutions that are up to 50% better than those we found by partial enumeration in Model 1.
In this article, we develop Model 3, which implements a variation of the random search method. We start with a solution (initially random), then swap the positions of a pair of devices. If that solution is better, then it becomes the incumbent solution and we repeat the process, otherwise we keep swapping positions in the initial solution. In effect, this process is a simple heuristic for searching the solution space around a solution. This method, known as a "local search", has the potential to improve the initial solutions. Will local search perform better than a pure random search in this situation?
23 July 2024
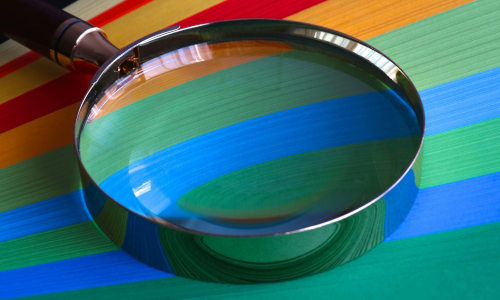
We continue our exploration of methods to solve our device rack positioning problem. The methods are:
- Model 1. Enumerate all possible position orders.
- Model 2. Search randomly for a specified time.
- Model 3. Local search around random starting positions.
- Model 4. Constraint programming using OR-Tools.
- Model 5. Mixed integer linear programming using Pyomo.
The previous article describes Model 1, which uses enumeration of all possible permutations. That method works well for a small number of devices, up to about 12. But it fails to find good solutions for larger data sets because it takes too long to run – up to tens of billions of years, for the larger cases.
In this article, we develop Model 2, which tries a parallel random search method. The idea is to randomly generate possible solutions for a specified time, reporting the best solution we find. This method has an advantage over enumeration: it samples from all parts of the solution space, unlike Model's 1 enumeration method which is limited by run time to a specific part of the solution space. But will Model 2 perform better than Model 1?
30 June 2024
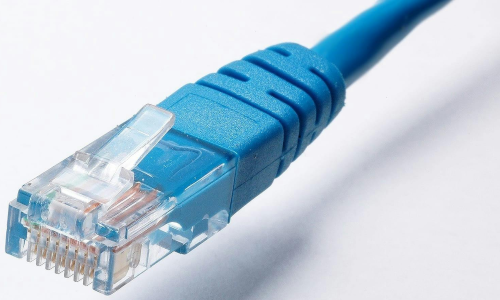
Most of the optimization models we work with involve finding the best combination of discrete decision variables. For example, allocating people to teams, designing warehouses, or scheduling staff.
A frequent question is, "Why not just look at all possible combinations and pick the best one?"
The answer is, sometimes, that's exactly what we do. In some situations, enumerating all feasible combinations of the variables is the easiest method for solving a problem.
But, in most situations, the number of combinations is too large. Many orders of magnitude too large. That's when we need more sophisticated methods, like mixed integer linear programming and constraint programming.
In this series of articles, we look at a simple situation that requires deciding the best order for positioning devices in a rack. We use five methods for solving this problem:
- Model 1. Enumerate all possible position orders.
- Model 2. Search randomly for a specified time.
- Model 3. Local search around random starting positions.
- Model 4. Constraint programming using OR-Tools.
- Model 5. Mixed integer linear programming using Pyomo.
Along the way, we see how a problem's size can quickly escalate to a colossal magnitude. We also demonstrate how, contrary to popular belief, that magnitude is not necessarily a barrier to finding a good solution.